How Fast are def
cdef
cpdef
?¶
Code Example¶
Here is an example of computing the Fibonacci series (badly) that will be done in Python, Cython and C.
First up, Python [Fibo.py]:
def fib(n):
if n < 2:
return n
return fib(n-2) + fib(n-1)
In naive Cython [cyFibo.pyx], it is the same code:
def fib(n):
if n < 2:
return n
return fib(n-2) + fib(n-1)
Optimised Cython where we specify the argument type [cyFibo.pyx]:
def fib_int(int n):
if n < 2:
return n
return fib_int(n-2) + fib_int(n-1)
In Cython calling C generated code. Here we use a def
to call a cdef
that does the body of the work [cyFibo.pyx]:
def fib_cdef(int n):
return fib_in_c(n)
cdef int fib_in_c(int n):
if n < 2:
return n
return fib_in_c(n-2) + fib_in_c(n-1)
Now a recursive cpdef
:
cpdef fib_cpdef(int n):
if n < 2:
return n
return fib_cpdef(n-2) + fib_cpdef(n-1)
Finally a C extension. We expect this to be the fastest way of computing the result given the algorithm we have chosen:
#include "Python.h"
/* This is the function that actually computes the Fibonacci value. */
static long c_fibonacci(long ord) {
if (ord < 2) {
return ord;
}
return c_fibonacci(ord - 2) + c_fibonacci(ord -1);
}
/* The Python interface to the C code. */
static PyObject *python_fibonacci(PyObject *module, PyObject *arg) {
PyObject *ret = NULL;
assert(arg);
Py_INCREF(arg);
if (! PyLong_CheckExact(arg)) {
PyErr_SetString(PyExc_ValueError, "Argument is not an integer.");
goto except;
}
long ordinal = PyLong_AsLong(arg);
long result = c_fibonacci(ordinal);
ret = PyLong_FromLong(result);
assert(! PyErr_Occurred());
assert(ret);
goto finally;
except:
Py_XDECREF(ret);
ret = NULL;
finally:
Py_DECREF(arg);
return ret;
}
/********* The rest is standard Python Extension code ***********/
static PyMethodDef cFiboExt_methods[] = {
{"fib", python_fibonacci, METH_O, "Fibonacci value."},
{NULL, NULL, 0, NULL} /* sentinel */
};
#if PY_MAJOR_VERSION >= 3
/********* PYTHON 3 Boilerplate ***********/
PyDoc_STRVAR(module_doc, "Fibonacci in C.");
static struct PyModuleDef cFiboExt = {
PyModuleDef_HEAD_INIT,
"cFibo",
module_doc,
-1,
cFiboExt_methods,
NULL,
NULL,
NULL,
NULL
};
PyMODINIT_FUNC
PyInit_cFibo(void)
{
return PyModule_Create(&cFiboExt);
}
#else
/********* PYTHON 2 Boilerplate ***********/
PyMODINIT_FUNC
initcFibo(void)
{
(void) Py_InitModule("cFibo", cFiboExt_methods);
}
#endif
Benchmarks¶
First a correctness check on Fibonacci(30):
$ python3 -c "import Fibo, cyFibo, cFibo; print(Fibo.fib(30) == cyFibo.fib(30) == cyFibo.fib_int(30) == cyFibo.fib_cdef(30) == cyFibo.fib_cpdef(30) == cFibo.fib(30))"
True
Now time these algorithms on Fibonacci(30) thus:
$ python3 -m timeit -s "import Fibo" "Fibo.fib(30)"
$ python3 -m timeit -s "import cyFibo" "cyFibo.fib(30)"
$ python3 -m timeit -s "import cyFibo" "cyFibo.fib_int(30)"
$ python3 -m timeit -s "import cyFibo" "cyFibo.fib_cdef(30)"
$ python3 -m timeit -s "import cyFibo" "cyFibo.fib_cpdef(30)"
$ python3 -m timeit -s "import cFibo" "cFibo.fib(30)"
Gives:
Language | Function call | Time (ms) | Speed, Python = 1 |
---|---|---|---|
Python | Fibo.fib(30) |
390 | x 1 |
Cython | cyFibo.fib(30) |
215 | x 1.8 |
Cython | cyFibo.fib_int(30) |
154 | x 2.5 |
Cython | cyFibo.fib_cdef(30) |
5.38 | x72 |
Cython | cyFibo.fib_cpdef(30) |
32.5 | x12 |
C | cFibo.fib(30) |
5.31 | x73 |
Graphically:
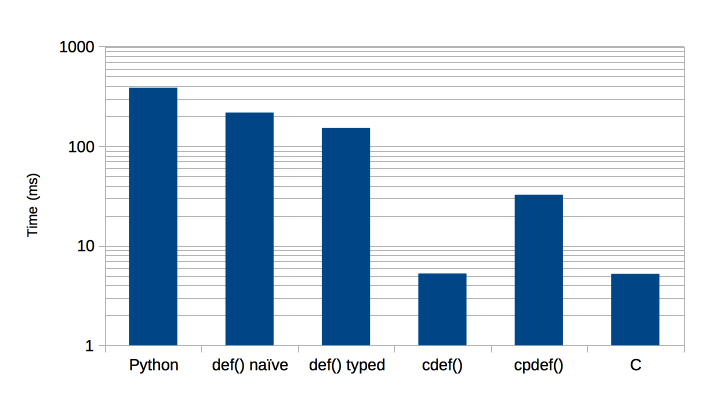
The conclusions that I draw from this are:
- Naive Cython does speed things up, but not by much (x1.8).
- Optimised Cython is fairly effortless (in this case) and worthwhile (x2.5).
cdef
is really valuable (x72).cpdef
gives a good improvement overdef
because the recursive case exploits C functions.- Cython’s
cdef
is insignificantly different from the more complicated C extension that is our best attempt.
The Importance of the Algorithm¶
So far we have looked at pushing code into Cython/C to get a performance gain however there is a glaring error in our code. The algorithm we have been using is very inefficient. Here is different algorithm, in pure Python, that will beat all of those above by a huge margin [1]:
def fib_cached(n, cache={}):
if n < 2:
return n
try:
val = cache[n]
except KeyError:
val = fib(n-2) + fib(n-1)
cache[n] = val
return val
And timing it for Fibonacci(30) gives:
Language | Function call | Time (ms) | Improvement |
---|---|---|---|
Python | Fibo.fib(30) |
390 | x1 |
Cython | cyFibo.fib_cdef(30) |
5.38 | x72 |
Python | Fibo.fib_cached(30) |
0.000231 | x1.7e6 |
Or, graphically:
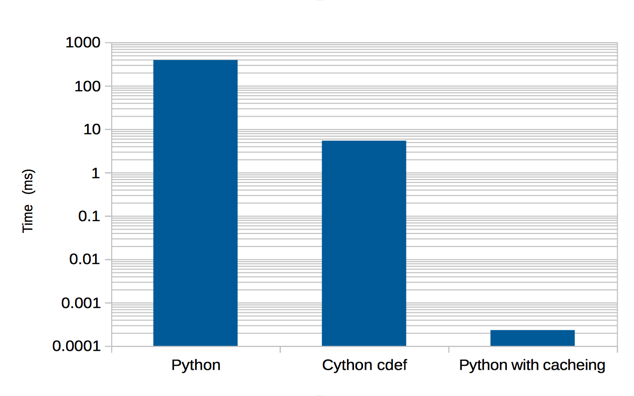
In fact our new algorithm is far, far better than that. Here is the O(N) behaviour where N is the Fibonacci ordinal:
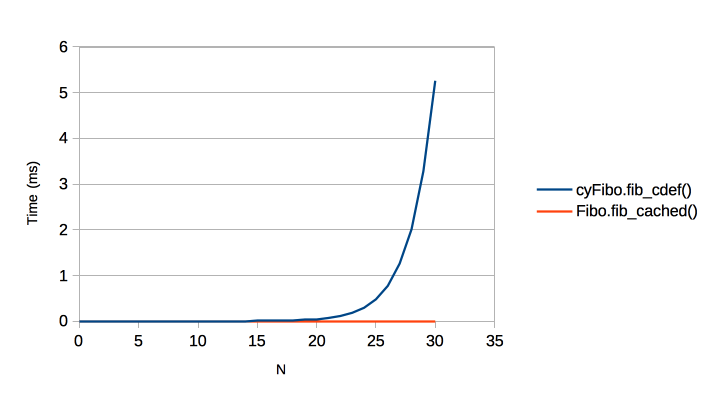
Hammering a bad algorithm with a fast language is worse than using a good algorithm and a slow language.
Footnotes
[1] | If you are using Python3 you can use the functools.lru_cache decorator that gives you more control over cache behaviour. |